The pharmaceutical industry has long relied on complex data and research to develop new drugs and treatment methodologies. With advancements in artificial intelligence (AI), the way we process and understand this data is rapidly evolving. One of the most promising developments is the application of LLM knowledge graph drug purpose. This emerging technology is poised to revolutionize how we discover and optimize drugs, helping researchers and pharmaceutical companies unlock new insights and improve patient care.
In this article, we will explore the role of LLM knowledge graph drug purpose, its impact on the pharmaceutical industry, and how it is transforming drug development and research. By understanding how LLM knowledge graph drug purpose is being integrated into modern healthcare, we can appreciate its potential for the future of medicine.
Table of Contents
What is an LLM Knowledge Graph?
To understand the significance of LLM knowledge graph drug purpose, we first need to break down the key components of this technology. An LLM, or Large Language Model, is a type of AI trained on vast amounts of text data to generate human-like understanding and responses. These models can understand complex relationships between words, concepts, and contexts in a way that was once thought impossible.
A knowledge graph, on the other hand, is a structured representation of information that connects entities—such as concepts, objects, or events—using relationships. It organizes knowledge into nodes (entities) and edges (connections), making it easier for AI systems to understand and retrieve relevant information.
When we combine these two elements into LLM knowledge graph drug purpose, we create a powerful tool capable of processing complex drug-related data. This tool not only organizes drug-related knowledge but also helps AI models understand the purpose and potential of each drug by analyzing how it interacts with other entities within the body, including proteins, genes, and diseases.
The Role of LLM Knowledge Graph Drug Purpose in Drug Discovery
In the realm of drug discovery, LLM knowledge graph drug purpose is helping researchers gain deeper insights into the potential applications of various compounds. Traditionally, drug discovery was a long and expensive process, often involving trial and error. However, the introduction of AI-powered systems has accelerated this process.
By leveraging large datasets of clinical trials, drug interactions, genetic information, and disease markers, LLM knowledge graph drug purpose can identify new drug targets, predict drug efficacy, and even suggest repurposing existing drugs for new uses. This allows researchers to pinpoint promising drug candidates much earlier in the process, reducing the time and cost associated with developing new treatments.
For example, by analyzing the relationships between drugs and diseases within a knowledge graph, an LLM can identify previously overlooked connections that could lead to new treatment options. Researchers can then validate these findings through experimental studies, ultimately bringing new drugs to market faster.
How LLM Knowledge Graph Drug Purpose Enhances Drug Repurposing
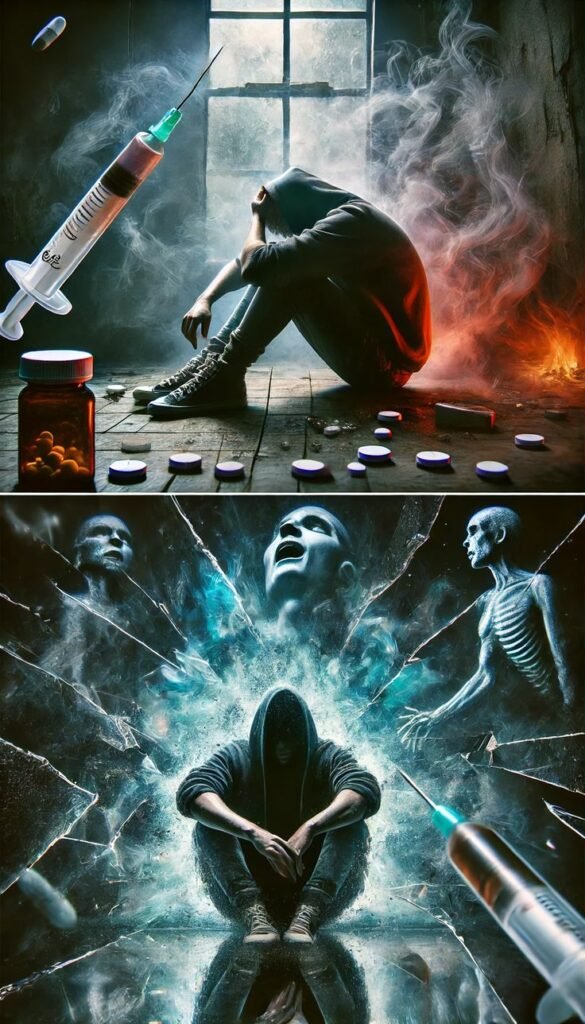
One of the most significant benefits of LLM knowledge graph drug purpose is its ability to assist in drug repurposing. Drug repurposing, or repositioning, involves taking an existing drug and finding new therapeutic applications. This is an attractive option for pharmaceutical companies, as it can dramatically reduce the time and cost required to bring a drug to market.
LLM knowledge graph drug purpose plays a key role in identifying potential candidates for repurposing. By analyzing vast amounts of medical literature, clinical data, and patient records, AI models can uncover novel connections between drugs and diseases that may not have been evident before. This can lead to the discovery of new uses for older drugs, offering patients faster access to effective treatments.
For instance, some anticancer drugs have been repurposed for use in autoimmune diseases, and certain antidepressants have shown promise in treating chronic pain. These findings were made possible through the use of AI-driven knowledge graphs, which help researchers uncover hidden patterns in complex data.
The Impact of LLM Knowledge Graph Drug Purpose on Personalized Medicine
Personalized medicine is an emerging field that tailors treatment plans to individual patients based on their genetic makeup, lifestyle, and other factors. The goal is to provide more effective treatments with fewer side effects by customizing therapies to each person’s unique characteristics.
LLM knowledge graph drug purpose is playing a pivotal role in advancing personalized medicine by enabling more precise drug recommendations. By analyzing a patient’s genetic profile and medical history alongside global drug knowledge, AI models can suggest the most effective drug treatments for that individual. This not only improves patient outcomes but also minimizes the risks associated with trial-and-error prescribing.
For example, if a patient has a genetic mutation that makes them more susceptible to a particular disease, LLM knowledge graph drug purpose can help identify drugs that target the specific molecular pathway associated with that mutation. This enables healthcare providers to prescribe drugs that are more likely to be effective for that particular patient.
LLM Knowledge Graph Drug Purpose and Drug Safety
Ensuring the safety of new drugs is a critical aspect of drug development. One of the challenges faced by pharmaceutical companies is predicting adverse drug reactions (ADRs). While clinical trials provide valuable safety data, they are often limited in scope and may not account for all potential ADRs.
LLM knowledge graph drug purpose is helping to improve drug safety by analyzing data from multiple sources, such as patient records, clinical trials, and real-world evidence. AI systems can detect patterns in adverse drug reactions by identifying relationships between drugs, side effects, and patient demographics. This can lead to the development of safer drugs and reduce the likelihood of harmful reactions once the drug is released to the market.
Moreover, LLM knowledge graph drug purpose can also assist in post-market surveillance by continuously analyzing new data as it becomes available. This enables pharmaceutical companies and healthcare providers to quickly identify and respond to emerging safety concerns, further improving patient safety.
Real-World Applications of LLM Knowledge Graph Drug Purpose
The application of LLM knowledge graph drug purpose is already yielding tangible results in the pharmaceutical industry. Several companies and research institutions are actively leveraging AI-driven knowledge graphs to streamline their drug development processes and improve patient outcomes.
For instance, companies like IBM, with their Watson for Drug Discovery platform, are using knowledge graphs to analyze the vast amounts of biomedical data available today. By incorporating LLM knowledge graph drug purpose into their systems, these companies are able to predict drug interactions, identify novel drug targets, and enhance personalized treatment strategies.
Similarly, startup companies and academic institutions are utilizing this technology to accelerate drug discovery and repurposing. By using LLM knowledge graph drug purpose, these organizations can explore a wide array of possible drug interactions and therapeutic uses that would have otherwise been overlooked.
The Future of LLM Knowledge Graph Drug Purpose
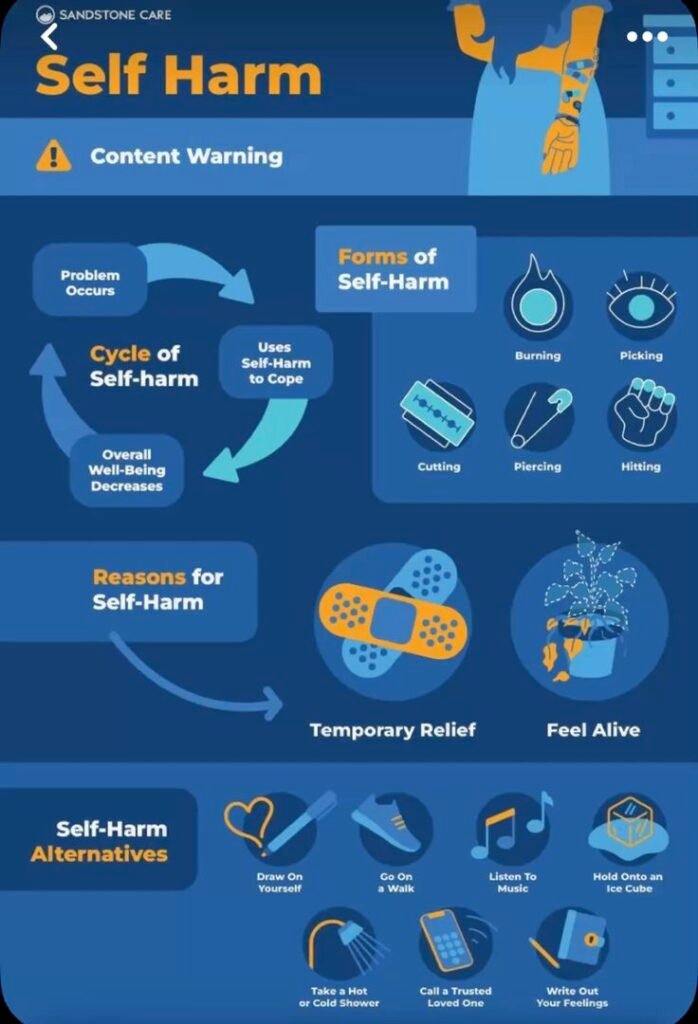
The potential for LLM knowledge graph drug purpose in the pharmaceutical industry is vast, and we are only beginning to scratch the surface of its capabilities. As AI technology continues to evolve, we can expect even more sophisticated applications of knowledge graphs in drug discovery, repurposing, and personalized medicine.
In the future, we may see LLM knowledge graph drug purpose being used to predict drug responses on a global scale, allowing healthcare providers to offer truly personalized treatment options to patients. Additionally, advancements in AI may enable more efficient identification of new drug targets, helping to combat diseases that currently have no effective treatments.
Furthermore, the integration of real-world data from electronic health records, wearables, and genomic sequencing could help improve the precision of LLM knowledge graph drug purpose even further. This could lead to more effective and targeted therapies, transforming how we approach healthcare on a global level.
Also read Did Trains Leave the Hurricane Path Earlu
Conclusion
LLM knowledge graph drug purpose represents a major leap forward in drug discovery and pharmaceutical research. By combining AI-driven language models with structured knowledge graphs, researchers are now able to uncover hidden insights, accelerate drug development, and offer more personalized treatment options. As this technology continues to evolve, it holds the potential to revolutionize the pharmaceutical industry, improve patient outcomes, and transform the future of medicine.
Through its ability to identify new drug candidates, repurpose existing drugs, enhance drug safety, and personalize treatment plans, LLM knowledge graph drug purpose is truly paving the way for a new era in healthcare. The promise of this technology is enormous, and its full impact has yet to be realized. As AI and knowledge graph technology advance, the possibilities for improving patient care and drug efficacy are boundless.